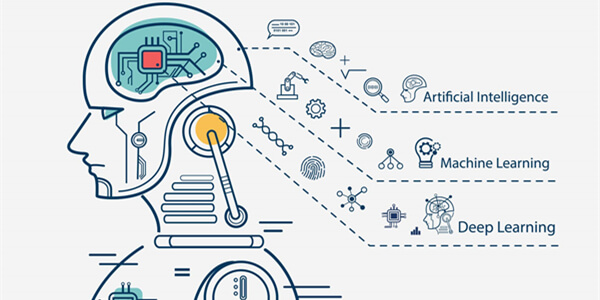
Transforming the Textile Industry: The Benefits of Data Mining and Machine Learning
I. Introduction:
The textile industry has been at the forefront of technological advancements, with innovations in textile manufacturing, dyeing, printing, and finishing processes. These technological advancements have increased textile products’ efficiency, productivity, and quality.
Despite these advancements, the textile industry faces challenges, including rising labor costs, environmental concerns, and changing consumer preferences. Technology has become increasingly crucial in overcoming these challenges and ensuring the sustainability and competitiveness of the industry.
In this context, this paper aims to explore the role of technology in addressing the challenges faced by the textile industry and how technological advancements can lead to a more sustainable and profitable future.
II. Why Data Mining and Machine Learning?
The textile industry generates vast amounts of data through various processes, such as production, supply chain, and customer interactions. Data mining and machine learning can help extract meaningful insights from this data.
Data mining involves using statistical and mathematical algorithms to discover patterns and relationships within data. On the other hand, machine learning uses algorithms that can learn from the data and make predictions or decisions based on that learning.
There are numerous benefits of using data mining and machine learning in the textile industry. These techniques can help improve product quality, increase production efficiency, optimize supply chain management, and enhance customer experience. For example, machine learning algorithms can predict product demand and help manage inventory. Data mining can identify defects in the production process and provide insights for process improvement.
III. Defect Detection
Defects in textiles can have significant implications on the quality and value of the product, leading to costly recalls and loss of consumer trust. However, detecting textile defects can be challenging, especially with the textile industry’s high volume and data variability.
Data mining and machine learning can help overcome these challenges by automating the defect detection process, allowing for faster and more accurate identification of defects. With the ability to analyze large amounts of data, machine learning models can learn to recognize patterns and anomalies associated with defects, enabling early detection and prevention of defective products.
Additionally, data mining and machine learning can improve defect classification and prediction, allowing manufacturers to identify the root cause of defects and implement preventative measures to reduce defect rates in the future. Using data mining and machine learning for defect detection, textile manufacturers can improve product quality, reduce costs, and enhance customer satisfaction.
IV. Color Matching
Color is a critical aspect of textile production, dramatically influencing consumer perception and purchasing decisions. However, achieving consistent and accurate color matching can be challenging, as variations in materials, production processes, and environmental factors can all affect color outcomes.
Data mining and machine learning offer solutions to improve color matching in the textile industry. These technologies can analyze vast amounts of color data, identify patterns, and predict optimal color formulations. This can lead to more efficient color-matching processes, reduced waste, and improved product quality.
V. Pattern Study
Patterns are an essential aspect of textiles, and they play a crucial role in designing and manufacturing textile products. However, pattern study poses various challenges, such as the complexity of patterns, time-consuming manual analysis, and inconsistencies in pattern recognition.
Data mining and machine learning techniques can aid in improving pattern study in the textile industry. For instance, computer vision algorithms can automatically recognize and classify patterns, reducing the need for manual analysis. Machine learning algorithms can also improve pattern-matching accuracy and reduce the time required for pattern recognition. Data mining and machine learning can significantly enhance pattern study and aid in creating high-quality textile products.
VI. Fabric Grading
Fabric grading is an essential process in the textile industry to ensure consistency in the quality of fabrics. However, this process can be challenging due to the subjective nature of human grading and the high cost and time required for manual grading.
Data mining and machine learning techniques can improve fabric grading by automating the process and reducing human errors. These techniques can analyze fabric attributes such as color, pattern, texture, and thickness and classify fabrics based on their quality grades. This approach can also lead to better product standardization and cost reduction.
VII. Applications of Data Mining and Machine Learning
Data mining and machine learning have various applications in the textile industry, including smart textiles, textile design, quality control, and supply chain management. Smart fabrics have advanced materials that can sense and react to environmental changes. Data mining and machine learning can be used to analyze the sensor data to optimize the textile’s performance. In textile design, data mining, and machine learning can be used to identify patterns and trends in customer preferences, allowing designers to create products that better meet their needs.
Quality control is an essential aspect of the textile industry, and data mining and machine learning can help identify defects and flaws in the manufacturing process. By analyzing data from sensors and quality control inspections, manufacturers can optimize their production processes to reduce the number of defects and improve product quality.
Finally, data mining and machine learning can improve supply chain management in the textile industry. By analyzing data from suppliers, manufacturers, and distributors, companies can identify bottlenecks and inefficiencies in the supply chain and optimize their operations to reduce costs and improve delivery times.
VIII. Conclusion
In conclusion, data mining and machine learning have numerous benefits for the textile industry. They can improve defect detection, color matching, pattern study, and fabric grading. Additionally, they can be applied to smart textiles, textile design, quality control, and supply chain management. The textile industry can achieve greater efficiency, accuracy, and profitability by embracing data mining and machine learning. As technology continues to evolve, there are endless possibilities for further advancements.